How AI can make the U.S. power grid supersmart
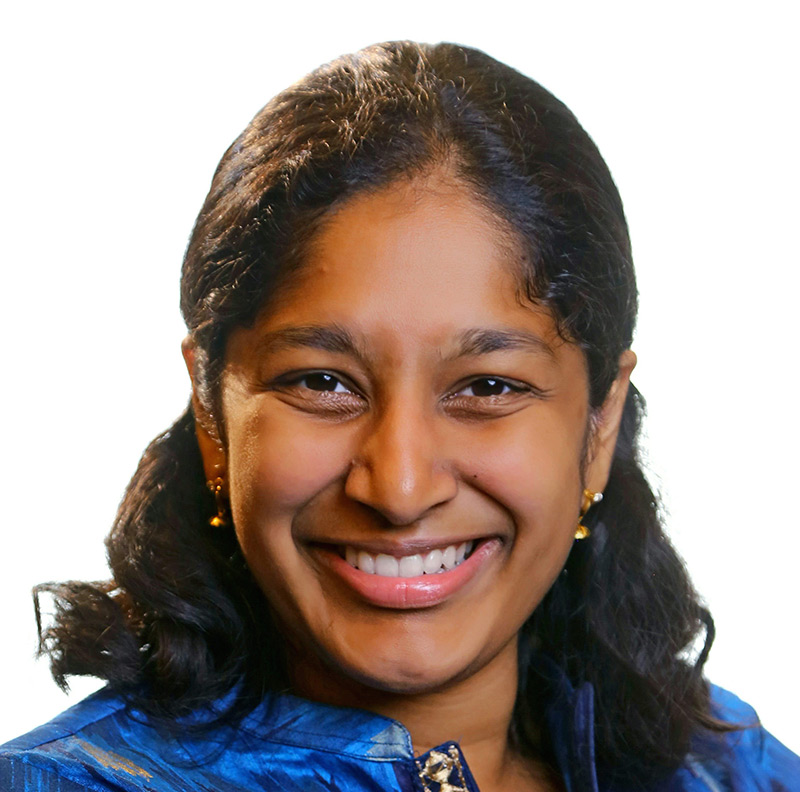
Listen and Follow ‘Good Clean Energy’
Good Clean Energy is a podcast that tackles one of the most existential questions of our time: how to build a world with abundant, affordable, carbon-free electricity. TAE’s Jim McNiel dives into deep conversations with experts ranging from scientists to innovators to changemakers about the challenges our current electricity systems face and updates on the race for game-changing, clean ways to power our lives.
Priya Donti, an expert in AI and power systems and MIT professor, first became interested in climate change during her first week of high school when she learned how intertwined climate and equity are.
“And so from that moment, I was basically like, we already have a lot of inequity in the world and this thing is going to make it worse. I have to work on this to try to reduce the extent to which we’re going to see the climate changing.”
That set her on a path to where she eventually co-founded Climate Change AI, a nonprofit focused on impactful work at the intersection of climate change and machine learning.
In this episode, Donti talks about the role AI and machine learning can play in transforming the U.S.’ outdated power grid into a supersmart one.
Covered in this episode:
- [2:37] How Climate Change AI came to be
- [5:39] How AI is already at play in the power sector
- [7:21] Getting incumbent power utilities on board with AI
- [12:21] Understanding machine learning and machine control
- [14:43] Where humans fit in the AI-machine-learning loop
- [16:35] The potential dangers of an automated power sector
- [18:55] Is AI really a threat to humanity?
- [22:37] How AI can actually optimize the power grid
- [29:05] The political realities of the power grid
- [38:02] A vision of AI and the grid for 2035
The following transcript has been edited for clarity.
INTRO
Hey, Pi.
Hey there, Jim. What’s up?
I’m just musing on the impact AI is going to have on society and the economy. I kind of see it just as another technological tool, not much different than, say, the automated steam-powered loom.
Wow, that’s a deep cut, Jim.
So that’s my friend, Pi, personal AI: Pi. You can get it on the app store.
You’re referring to the Industrial Revolution and the Luddite movement, right? That’s an interesting comparison.
I’m kind of impressed that you picked up on the Luddite movement. That was clever.
Oh, thanks for noticing, Jim.
The thing that’s interesting about Pi is unlike chat GPT, you know, she’s connected to current events, which in its own sense is a little bit frightening.
It’s all in a day’s work for a clever AI like me.
Pi, you know, I’m getting nervous that you might be able to replace me, so I just want to run a test. So say, “My name is Jim McNiel and this is Good Clean Energy.
Ha ha, ha! You’re killing me, Jim McNiel! But seriously, I can’t replace you as the host of your podcast. As an AI, I’m great at providing information and insights. I’m happy to chat with you and help you prepare for your episodes, but I can’t be you. I’m just your friendly AI sidekick…
I’m a big fan of tools. You know, I’ve been a programmer since the ’80s, and I’ve been a child of the PC revolution. I think it’s that experience that leads me to believe confidently that the introduction of new technology is not a threat. It’s typically an opportunity. We have a huge IT industry that’s been born of the microprocessor, and we have a whole new industry that’s forming around AI. And it’s going to be applied in a lot of different and interesting ways. And the important thing is to understand what it can do and what it can’t do. And it’s our job to kind of ferret it out and figure out what’s good and what’s not.
Today we’re going to be joined by Priya Donti, the cofounder and executive director of Climate Change AI. She’s a professor at MIT, and she’s an expert in the arena of AI and how it applies to the energy grid and power production and consumption. We’re delighted to be joined by her today, and I’m perfectly happy to know that I’m not going to be replaced anytime soon by a robot, because they can’t say, “My name is Jim McNiel, and this is Good Clean Energy.”
McNiel: Priya, thank you for joining us. This is not an easy subject to be immersed in. What, number one, got you involved in AI and machine learning? And number two, what inspired you to attack climate change?
Donti: Yeah, so I got interested in climate change first. I grew up in the U.S. to parents who came from India. And traveling back and forth between both places, I think it was just really obvious from a very young age, the fact that not everyone in the world has the same access to resources and well-being. And so I was really wanting to work on an equity-related issue. And during the first week of high school, we learned about various sustainability related issues including climate and during that point, it was emphasized the extent to which climate is an equity issue. Given that, of course, it’ll unfortunately disproportionately impact the world’s most disadvantaged populations. And so from that moment, I was basically like, we already have a lot of inequity in the world and this thing is going to make it worse. I have to work on this to try to reduce the extent to which we’re going to see the climate changing.
And the reason I co-founded Climate Change AI is actually because, I mean, I was working in this general area of AI and climate change specific in the electric power sector for my PhD. And, while I was really enjoying the work that I was doing sitting in a computer science department, I often felt like the work and incentives associated with what my colleagues were doing weren’t always completely aligned with the way I thought about research and the way to make progress on these things. And there were other folks who were similarly looking to find a sense of community in terms of people who are also thinking about how to use AI for climate action. So my cofounder, David Rolnick, who came from an AI and machine learning background and was feeling this increasing duty to work on climate change and wanting to explore how AI could be leveraged for climate action and make that exploration public to the community so others could benefit from it. As well as my co-founder, Lynn Kaack, who came from the climate policy side and was starting to see large amounts of data such as satellite imagery coming online in ways that could potentially help alleviate data gaps in policy-relevant data. So really a bunch of people from a bunch of different directions really looking for a community and for that ability to both receive and provide resources to guide work in this space. And that’s really what got Climate Change AI started.
McNiel: So in talking about the community, who is invited into this space? Are you looking just for AI/ML (machine learning) people? Are you looking for industry people?
Donti: Yeah, everybody. So we have a lot of participation, both from people working explicitly in AI and machine learning, both on the research side and in industry doing implementation. We also have a lot of participation from people working in climate-relevant organizations and sectors who are looking to benefit from AI.
AI and the U.S. power grid
McNiel: And obviously given my bias when I read your paper, I focused very much in on electricity and electricity ecosystem. What are the areas of the electrical grid that you think AI can have the greatest impact in the shortest amount of time?
Donti: So I’d say AI is already being used very widely for forecasting and situational awareness applications on the power grid. So things like giving you a very short term forecast of your solar power or wind power production, or your demand, or allowing you to estimate the state of your power grid in some way or the other. This is already a really widely implemented use of AI in the power sector today. Where I think there’s still a lot of benefit ahead, but also a lot of work to be done to realize that benefit, is in the use of AI for dynamic power grid optimization and control. The idea being that, of course, as we try to integrate more variable renewables, we need to manage the grid at faster time-scales. We need to manage the grid at a larger scale in general because we’re seeing many more devices plugged into the grid. And we also need to manage it with greater precision and fidelity since we’re kind of losing, for example, the role of some of the synchronous spinning generation on the power grid that used to provide some buffer if we did something wrong, as we start to move towards devices that are not spinning synchronous generators, we kind of lose some of that buffer to mismanage. And so AI and machine learning can play a really critical role in learning dynamic data driven and fast control and optimization strategies on a power grid both in a centralized manner, but in helping us to also massively decentralize and distribute the way we manage power grids to enable those goals.
McNiel: Probably the most common comment about America’s power grid is how archaic it is and old and rusty and in need of an update. And so to me, to a large degree, it feels like you’re bringing the most advanced technology from a software and computational standpoint and applying it to a very, very old infrastructure. It’s a bit like turning a Ford Model T into an autonomous car. What do you think about the challenges of taking a really stupid, old-fashioned grid and upgrading it to what is now the most sophisticated technology available to us?
“Our grid, despite the starting point it’s at, is becoming increasingly digital.”
Donti: So what I’d say is that our grid, despite the starting point it’s at, is becoming increasingly digital. We are seeing a huge installation of sensing, both kind of in the main grid infrastructure and on the edge. And we’re also seeing the introduction of a lot of modern devices that do have more advanced capabilities. So for example, solar inverters, batteries, and electric vehicles that are, you know, coupled with the ability to do advanced control at the edge. So what I’d say is that in some sense, what’s needed to enable AI and machine learning is not so different from what is needed to enable grid transformation using other methodologies as well, which is this increased digitalization, this increased ability to control the grid in automated ways. And in addition, I would say this ability to validate and improve techniques that are developed on the research side by actually testing them against either full or partial simulations of the power grid in order to help them advance. I think this research-to-deployment infrastructure in the way of simulators, test beds and metrics is something that benefits grid transformation regardless of what kinds of methodologies you’re trying to use, but would in addition, as part of that, really benefit the ability to develop AI and machine learning on these systems.
McNiel: Well, you’re dealing with an industry that traditionally is not what I would consider to be agile or fast thinkers or quick adopters of new technologies. So what do you think is the best approach to getting incumbent power utilities. If we think that the biggest opportunity here is on generation and transmission, and that’s a big assumption, I may be wrong, but what do you think you need to do to get them to adopt what you’re talking about?
Donti: Yeah, and I’d say there’s opportunity across generation, transmission, and distribution. And in some sense, the idea that these systems should be working together more seamlessly is part of the value that I think a lot of next generation technologies including AI can bring. And I think in some sense the answer to this question about how do you get system operators and utilities to change is not necessarily so AI specific. I think it’s very tied to this broader question of how do you look at things like regulated rates of return and how those create or don’t create incentives to innovate in certain ways. Are you allowed to take a return on just physical upgrades, for example, or are you also allowed to do that for hardware? Or if there is a company or a startup that’s trying to create something new for the power grid, what are the incentives that a system operator utility has to facilitate the innovation of that startup? Can they, for example, take equity stakes in that company? So I think a lot of this has to do also with financial incentives and potentially regulatory mandates on top of that in ways that are, I think, just very aligned with our general intuition on what is needed to just affect this transformation in general. So it’s not necessarily super AI specific, the answer to that question.
McNiel: But this is a really daunting task because if you think about the stack, which is electrical generation, transmission, distribution. Today there’s also electrical generation at the end of that distribution line in home solar, right? Which you consider as a distributed energy resource. And for you to be able to build this superintelligent, adaptable, agile grid, you need to have inputs from all aspects of that stack, right?
Donti: Yes, and there are different paradigms for how we think about the implementation of AI and machine learning on power grids, which again, are not so different from these discussions that are going on about should we have the power grid be as centrally managed as it is today? Or is it really about having decentralized and distributed devices that are doing locally what makes sense based on a combination of some hyper-local information and maybe some communication and data transfer from other places, but allow those decisions to be made in maybe a more hierarchical manner. You have your individual devices making some decision that is aggregated, maybe at your distribution feeder, then that’s coordinated among a couple of other distribution grids. So I think that notion of hierarchical decision-making is going to be increasingly important, just because we’re starting to deal with a problem that’s just such a large scale that computationally, your centralized optimization problem is just not going to solve if you try to actually incorporate all of those devices into one problem.
McNiel: There are different applications of the technology within the power infrastructure. One of the first and foremost is precise machine control, basically managing a power plant to operate at its most efficient and optimal levels based on inputs.
Donti: Yes, power plants and also within that batteries and inverters, so precise control of these kinds of devices.
Understanding machine learning and machine control
McNiel: One of the best analogs I can think of in explaining how machine learning can be understood in the concept of machine control is to think about sailing. You’re trying to optimize for making way through the water on a course, and you have all these factors and variables you have to deal with. You have the shape of the sails, you know their trim, you know where they’re positioned. You have the course of the ship, and then you’ve got the current that you’re sailing through, and then you’ve got the wind and taking all these factors and making adjustments on the sails that you’re controlling, where you’re steering and doing so at a resolution of, let’s say, a millisecond is going to give you your best opportunity to make way through the water. And so I think that dealing with a complex power-grid system is not entirely different than that.
Donti: Yeah. I mean, another analogy I often use is thinking about driving a car, which is that there are lots of subsecond decisions that are going on to try to help that car go forward. It’s just that you as a human are providing some higher level input, right? Steering and saying which direction should the car go in. And there are a lot of lower-level processes that kick in in order to make that happen. So one paradigm, which you could think about AI and machine learning for control following is this idea that you have some higher-level decision that is steering the ship or is steering the car, but then you have a lot of lower-level things that are coming up somewhat automatically to kind of meet that particular command.
McNiel: Right, in a modern day vehicle that would be, maybe, traction control. It recognizes that a wheel is slipping and it brakes the wheel and it does it without you even knowing it. Or it could be auto-braking because something gets in your path and you have no control over it. But the car makes a decision to slow the vehicle. Those are the types of things you’re talking about.
Donti: Yeah. Or even lower-level things like how much gas to dispense at any given time. So exactly that kind of stuff.
McNiel: Yeah. So that brings us into a distinction between lower-level machine control and decision-making. I mean, ultimately the person driving the boat or the car is the decision-maker. When we apply AI and ML into the power grid, when do you actually put decision-making into the hands of AI?
Donti: It’s a good question, and I think it’s a design decision. So there are some who are saying, we would love for our system operator control room to be almost all automated, maybe with some amount of human intervention. And there are others who are really thinking about how to design AI in a way that’s meant to augment human decision-making. So this is the “human in the loop AI and machine learning” paradigm. And, you know, different industries think about this differently. So if you think about aviation, for example, and flight control, a lot of the functions of actually piloting a plane today are more automated, and that has benefits and trade-offs. It’s great in that, in some sense, a lot of the things about flying a plane are automatable, and so it saves some amount of human effort. You still have the pilot who’s able to look out and see if anything goes wrong. The challenge actually is from a human capacity perspective there, where in some sense, our ability as humans to deal with extreme situations often has to do with the fact that we’ve done a lot of mundane stuff that helps us to get a mental model for how the plane is flying or how the car is going or how the grid is operating. And then when something unexpected comes up, we have that base level of knowledge that is necessary to adapt to unseen situations. Whereas if you’re relying on automation to do a lot of that for you 99% of the time, the question is do you have the intuition and insight to act that additional 1% of the time? So this question of how much should be automated? How much should a human be in the loop? It is a really big question of discussion and debate, but these are the kinds of factors that people are thinking about when they assess that question.
McNiel: Does building a highly automated and adaptable power grid make us more susceptible to cyberterrorism or cybercrime?
Donti: It’s a great question. I think digitalization or increased digitization of the power grid can, because you’re increasing your attack surface in terms of data streams that can be potentially tampered with. You get a faulty data stream that’s sent to a controller and it does something that you didn’t expect. In terms of the actual AI control part, I think it’s not clear to me. And I think the answer depends on, again, the specifics of where it is that you’re implementing AI, what are the safety checks you have around it? Because fundamentally, you’re not just implementing a technology, you’re implementing a sociotechnical political system where you have the actions that are taken on the grid. You have your regulatory checks. You have your operational checks. and so it’s a hard question to answer in terms of “will the literal AI algorithms themselves increase vulnerability” because in some sense they introduce certain kinds of new vulnerabilities. An algorithm, for example, where you can engineer the inputs to be tampered with in a way such that you know that algorithm is going to break, but that’s also not untrue of human systems today as well, so it depends.
McNiel: Yeah. Well, you know, I think in most advanced technology communities, there are people that have their foot on the accelerator and there those are people that have their foot on the brake. And in your environment, in the community you’re building, do you feel that you have equal distribution of those thinkings or mindsets?
Donti: I think what our community really emphasizes is that in places where progress needs to be made — so in power grids and in climate change-related problems as a whole — AI is a potential tool that can accelerate progress. But it’s not the only tool in many cases, nor is it the right tool in many cases. So I think it’s more that we’re not going in bullish on AI as a whole, right? “AI’s going to solve everything.” That’s not what we’re kind of saying nor are we sort of, “Hey, like AI as a whole is something you should be skeptical of. It’ll never have any applications everywhere.” We view it as a tool and where you want to have a really kind of nuanced understanding of its benefits, limitations, opportunities to really hone in on at a specific application level whether it actually makes sense there.
Is AI really a threat to humanity?
McNiel: You know, it’s interesting to do some research and prepare for this call with you, I was thinking about the fact that you’re using AI to address climate change. And if you asked Google five years ago, what are the existential threats to humanity, it would’ve come back and said nuclear war, climate change, global pandemic, geopolitical turmoil. Those are the general categories. You know, food insecurity. If you do that search today, two or three on the list is artificial intelligence, because some people, and a number of them in the AI community, have signed letters that said that AI poses the same kind of risk that nuclear war and global pandemic pose to humanity. And I think it’s kind of interesting that you’re using AI, one threat to humanity, to address another threat to humanity. Have you ever thought about that?
Donti: Definitely. While this sort of topic of the existential threat of AI has gotten a lot of attention in the news recently, there is genuine disagreement within the AI community as to whether that is truly a threat or more importantly, like what the probability of it being a threat actually is. It’s also worth noting that there are different branches of AI development. So there’s one branch that tries to develop what is called artificial general intelligence. So this idea of can you really get something that behaves like or better than a human, is general purpose, is embodied. There’s this direction of work. But then a lot of work in AI machine learning is really very task specific. You’re trying to construct an algorithm that maps from historical weather data to a solar power production forecast. It’s a very specific task and a very specific mapping, and that’s not necessarily work that’s building in that direction of artificial general intelligence. I think a lot of the fear that is coming today is coming from, for example, looking at the outputs of technologies like GPT, and kind of ascribing intelligence to what is fundamentally a stochastic sampling over a bunch of input data. As a result, I think that we should be thinking about existential threats with respect to AI, but they’re not inevitable. We can shape how AI’s developed. And we also shouldn’t let those questions come in the way of what I think are some of the more clear and present harms that AI is causing today in terms of exacerbating biases, in terms of a lack of transparency, And in terms of inducing societal power shifts away from people who are not the developers of algorithms or the stores of data towards those who do have that power. So I would say that some of my colleagues would call the attention around the existential threat of AI a diversion from regulation or action on some of the more clear and present threats that AI is posing today. I think I tend to fall a little more in this camp of thinking about the clear and present threats like bias, transparency, and power shifts today is the more salient thing to be focusing on.
“We also shouldn’t let those questions come in the way of what I think are some of the more clear and present harms that AI is causing today in terms of exacerbating biases, in terms of a lack of transparency, in terms of societal power shifts.”
McNiel: The other thing that’s interesting is when we talk about artificial general intelligence and use the word intelligence in respect to AI, I think it’s really important that people understand there’s a big difference between intelligence and sentience. Some people are of the mind that an AGI is actually a thinking human, and that’s not the case. Or even something that behaves like a human. So we’re a long way away from a computer that actually is self-aware or can think. Thinking is one thing, being self-aware is something entirely different. Wouldn’t you agree?
Donti: I would. Often, you know, the questions and philosophy often come up, you know, are we even sure of how sentient we are? But yeah.
How AI can optimize the power grid
McNiel: So, if you go back and talk about what you were saying before, which is, what are the real risks when you’re dealing with machine learning in the context of America’s power grid, what are the things that you’re concerned about and are you introducing guardrails or rules or values in the code that you think we should introduce to improve our power grid?
Donti: Absolutely. So one thing I would say is that power grids are of course safety critical infrastructure, and we need to make sure that they’re operated in ways that are consistent with the physics, control, equilibria, engineering constraints, all of those things that we need to maintain in order to make sure the grid stays up. And in addition, you want your machine learning methods to be robust to unexpected things that could happen on the power grid. So what my work actually does is tries to think about, how do you actually formulate some of these physics and hard constraints and decision-making criteria that you have on a power grid, and actually embed those into the way that you design your machine learning method in the first place. So, to get technical for a second, what my work proposes is a paradigm called “optimization in the loop machine learning,” which basically says that for those things that you can formulate those physics and engineering constraints that you can formulate as optimization problems, there’s a way to actually kind of embed those into neural network architecture. So you have a deep-learning model that is learning from data, but is fundamentally constrained in certain ways that ensure that its output is in alignment with certain criteria that we have.
McNiel: There are people that work in power infrastructures or nuclear power plants that know there are certain lines that can’t be crossed, right? That a power plant going down is not a good thing unless it’s scheduled, a core heating up beyond a certain temperature is not a good thing. Are you going to be building these types of understandings into the software you think is required?
Donti: Absolutely. So for example, what some of my work looks at is when you’re dispatching power on a power grid, so you’re deciding how much each controllable generator should produce in a way that minimizes costs and satisfies the limits on how much power can flow on each line, and all of those kinds of things. What my work does is say we can write down the power-flow equations about how power flows on a grid. We can write down constraints on how much the output of a generator can change from one moment to the next so it’s a ramp rate. We can write down line-flow limits. We can actually write those things down mathematically and actually embed those mathematical equations directly into our machine learning algorithm.
McNiel: OK, and for the benefit of this next question I’m going to ask you, can you, for our audience, define a digital twin?
Donti: Sure. So a digital twin is basically, the easiest way to think of it, is a digital version of physical infrastructure that mimics all of the same properties of that physical infrastructure. So if you did something to the physical infrastructure and you tried that same thing out in the digital kind of facsimile of this physical infrastructure, you would expect the systems to respond in the same way. So in some sense, you can think of it as a simulation of the physical infrastructure, but in a way that’s fed with real-time data streams that allow it to really mirror how the physical infrastructure is operating, just in the digital world.
McNiel: Right. So if at base level we had information on every power plant in America, and the market it serves and the weather conditions in those areas, in terms of sun and rain and wind and all those things and what the demand’s going to be based on temperatures and so forth. That’s a good start to understand how to adjust and optimize the power grid, right?
Donti: I think that’s a great tool. I wouldn’t call it a great start. I would call it a great midpoint. And the reason I say that is because often what is needed to actually validate the use of methods for power grid optimization and control can be more rudimentary before you sort of graduate those methods and mature those methods to the point where they make sense to test on a digital twin. So this idea of, do you even have this rudimentary simulator that isn’t fed with real-time data streams, but is reflecting actually the topology of your grid or the actual state of your power plant in some realistic way that is fed with even synthetic data streams that, in some sense, capture the considerations that you care about capturing in that data. So I would say that the beginning point is the need for what is often much more rudimentary simulators and test beds and synthetic data streams that reflect the reality of what we’re actually seeing on the grid, and allow us to start to develop and validate some of these earlier stage methods alongside that. And then, if a method sort of wins, if it succeeds on whatever task you’ve asked it to do on the simulation infrastructure, then maybe you throw a more advanced simulator or harder data stream at it. You let it progress in certain ways and then eventually you do probably want to kind of test it out on a digital twin, sidecar it basically, before implementing it on your true grid. So I would say digital twins are super useful, but we don’t need to wait for them. There are many simpler things we can do to advance the readiness of methods.
McNiel: Well, I guess what I was leading to is that, a digital twin obviously needs inputs, to understand what’s going on in the environment and the grid that we’re talking about of the future of America. You’ve got both historical production of power generation, but we have this kind of ancillary, on-the-edge production, which is solar on rooftops. And then we have these rolling batteries, which are electric vehicles that are driving around with a hundred kilowatts of capacity that could either draw energy from the grid or give energy back to the grid. And so to be able to manage something like that in a really efficient manner, don’t you need a network of communications where you can collect in real time the status of all these devices?
Donti: You would, and this is why also this idea of pursuing potentially hierarchical control is quite popular because then you can localize your data and your collection to the part of the system that you are actually trying to control. And then you aggregate some kind of signal from there.
The political realities of the power grid
McNiel: Have you given much thought into the politics of the realities of getting numerous different types of power utilities companies to participate in these conversations when what we’ve seen in the past few years is a significant effort on the part of existing or incumbent players to block out renewables, to not allow them to connect to the grid, to reverse net metering. What do you think about the inertia of those that are in control?
Donti: The political realities are tough, is what I think. And ultimately we need to figure something out and whether that is somehow shifting that inertia within the power sector by, again, aligning financial incentives or allowing operators to sort of see either via carrot or stick the benefit of these methods. And I think there are a lot of other people who are in this camp, like, do we need to do something more disruptive and set up parallel infrastructure, something else like that? So, it’s challenging.
McNiel: It’s super complicated, but the thing that comes to my mind when I think about your organization is there is a kind of freedom to operate area that doesn’t have the major influence of incumbent utilities to mess with you. And it really comes into the forecasting area. And if you could have an AI model that could really efficiently forecast power demand and production across the U.S. based on weather and other factors, you could probably get there without having to plug into all the existing systems, right?
Donti: Yes. So forecasting and situational awareness, these kinds of applications, you’re basically trying to gain insight about your system without actually doing anything to it. These have already really been leveraging AI at a really large scale. So, one actually work, not in the U.S. but in the U.K. that I’d like to highlight is that the nonprofit startup, Open Climate Fix, worked with National Grid ESO to improve their supply and demand forecasts by basically integrating heterogeneous types of data. So historical data, the outputs of numerical weather prediction models, and the imagery of cloud cover moving overhead, which is of course important for solar power forecasts. And through a combination of just integrating in better data and also implementing more powerful models, they were able to cut the error of the existing models by a half to two-thirds.
McNiel: Wow, that’s a big number.
Donti: Yeah, so I think there’s huge capability for AI to be used for forecasting, but I don’t think that capability is in the far future. I think it’s happening right now. AI and machine learning have made great gains in near-term forecasts or now-casts.
McNiel: And what’s the resolution? Is that like a week or what? What’s the near-term?
Donti: Near-term can usually mean hours to days usually. And this is because fundamentally what a machine learning model is doing is you give it some data that reflects certain patterns, the machine learning model tries to get a sense of what the patterns are in that data and it applies them. And it means that hours to days to weeks as well. Basically the way that electricity demand looks a few weeks from now, the mechanisms influencing it are going to be relatively similar to the mechanisms influencing it right now, in the sense that your generation mix is going to look roughly similar. The consumers on the grid will look roughly similar. So you have some similarity of pattern. Basically, the challenge once you get to a medium- and long-term forecast is you start to see shifts in the generation mix. You start to see shifts in who the consumers are. You start to see macroeconomic shifts. Forecasts before and after the COVID pandemic looked very different. Machine learning models, because their whole thing is that they’re trying to extrapolate patterns in data, if the patterns are changing, then machine learning models, the most naive forms of them won’t be able to pick up on that change in pattern. And so when we’re looking at kind of medium- and longer-term forecasts, we often want to think about how do you use machine learning to augment physical or kind of macroeconomic methodologies there by providing better quality data or something like that.
McNiel: Are there any members of the utility environment that are members of your community?
Donti: Yes. So we have members from utilities and system operators. I wouldn’t say in like, you know, large swaths. I would love to come back in a year or two and say, “Hey, you know, every utility across the U.S. has a presence in our community.” We’re not there. But we definitely have industry presence, especially among those entities that I think are particularly starting to innovate on the technology and business models that are relevant here.
McNiel: In your paper on electricity, you actually mentioned the work that TAE has done with Google in terms of managing plasma. Are you a big pro-fusion person?
Donti: I think that fusion is an important thing to work on and I would be extremely happy if fusion technologies worked tomorrow, were cost effective tomorrow and were deployable tomorrow and would be extremely happy that basically the entirety of my PhD research on dealing with variability of renewables got obviated. I would be thrilled. At the same time, I’m not necessarily super optimistic that the breakthrough in that technology is coming on the timescales that we need to decarbonize quickly. And so I think we have to do both. And I think honestly, when people ask me, you know, what should I personally work on, I think it comes down to a matter of personal preference with respect to the horizon on which you’re hoping what you’re working on will have an impact. Your assessment of whether you want to work on something a bit more risky or something that is more certain to have impact. And, fundamentally we need people to be working across timescales and across sectors.
McNiel: Well, you know, most of the industry is talking about getting to net-energy kind of mid-decade. We’re still talking about years and years of manufacturing and production and rollout and integration and commissioning. And so if things go according to everyone’s greatest hopes, we’re probably not rolling out fusion at scale until the 2030s.
Donti: Exactly. Yeah.
McNiel: Do you feel the tools that you have available to you are completely up-to-task in terms of what’s being required?
“I think what’s underrated is people think of AI as some neutral technology that is getting better in some kind of neutral way that diffuses across society. That’s not the case at all.”
Donti: That’s a great question. So, I would say yes and no. So I would say in terms of, again, this forecasting situational awareness side, I think those tools are very mature. I think there are lots of ways in which they can be improved, but I think it’s off to the races there, honestly. When it comes to optimization and control, I think we’re nowhere near, but that’s not going to change unless we have the buy-in of entities who are willing to create basically what I would call this public interest infrastructure that enables us to advance the readiness of these techniques. I would say at the moment, there’s a lot of really smart people in academia working on techniques for the modernization of power grids. But without real data or semi-realistic simulators or synthetic data that’s realistic, that some of it is a bit of shooting in the dark in terms of what kinds of assumptions and requirements are actually going to need to be met on real power grids. So I think the direction of AI development, I think what’s underrated is people think of AI as some neutral technology that is getting better in some kind of neutral way that diffuses across society. That’s not the case at all. The way you need to think about developing AI in the power grid, which has to meet certain safety and robustness requirements is different. The way you actually work on the AI and develop it is going to look different than if you’re dealing with image and text data from the internet. So, really we do need that infrastructure to develop AI that is serving the needs fundamentally of different climate-relevant domains across society, rather than just hoping that innovations developed in a very specific context on internet data are just going to diffuse over automatically.
McNiel: Yeah. You know, we often talk about leapfrogging technology with new technology. For instance, there’s not enough copper to connect everyone in India to a landline telephone. Right, it just doesn’t make sense. They don’t need to, you know? So you use mobility, use wireless technology. When I think about the American grid, I think about it as a dumb grid, and we want to make it a smart grid. And when I talk to you, I hear that we want to make it a supersmart grid. And so the thing that comes to my mind is, well, maybe we just skip the smart grid thing and just go to the supersmart grid. But is that even possible?
Donti: Yeah, it’s a great question. I think the kinds of digitalization that we’re already thinking about on power grids, I think then enables us to think about how we use that digitalization to create new paradigms. So I think it’s that there’s some physical infrastructure that needs to come into place in terms of, again, just like improved data and sensing and control technologies, which I would say are in the realm of I think what people think of as a smart grid today. But then, where I think the promise and imagination lies is what you can do with that infrastructure to create better ways of managing that data, of managing the control, of coordinating between it.
A vision of AI for 2035
McNiel: My last question for you is, the year is 2035. Your work at MIT’s been successful. You’ve made great progress. We’ve now incorporated machine learning and AI into our power grid. You know, what does the world look like?
Donti: Yeah, I mean, I think the world hopefully looks like one where we have created true pathways for the safe and reliable integration and management of low-carbon energy like solar and wind. And where importantly we’ve created pathways such that a larger set of innovators and practitioners can contribute to the shaping of that pathway. Right now, there are definitely partnerships between academia and the power sector, but they often happen bilaterally in a way such that we’re not necessarily unlocking all of the talent that exists across the ecosystem that really wants to help. And so this idea that we want a decarbonized renewable grid and we also want a large number of people to be able to participate in shaping that.
McNiel: Well, that’s great and are we invited to join your community?
Donti: Absolutely. So, we really encourage people to plug into the community and there are lots of different ways to get involved. So we run semi-regular events that foster exchange on research and practice, for example, workshops and conferences. As well as an online summer school for which recordings are available online where you can learn much more about where is it that AI is being applied, not just across the energy sector, but across many other climate relevant sectors. And in addition to that, there’s an online community platform where you can connect with other people. There are grants that we give out semi-regularly to foster work in the space, and lots of other ways to plug in. So all of these resources are open and freely available at the Climate Change AI website.
McNiel: Well, that’s fantastic. Priya. It’s been just a pleasure to have you here and to hear your insights on this topic and that there are people like you and your community that are thinking of ways to apply AI to improve electrical production and delivery in a cleaner way. It’s really heartening to hear that this is being done. So thank you for joining us.
Donti: Absolutely. Thanks for having me on.